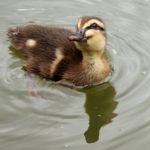
/
RSS Feed
Old prejudices are often coded into new technologies, even those technologies that claim to enhance diversity and fairness. We break down the metaphors of the New Jim Code (from Ruha Benjamin) and the Digital Poorhouse (from Virginia Eubanks) to show how modern technological “fixes” discriminate against Black people and poor people, respectively. Even the best-intentioned algorithms can have disastrous consequences (not unlike Abi’s cooking). We suggest some ways that designers and communicators can better account for race and poverty in their designs. In addition, we reveal the fourth rhetorical appeal from Aristotle’s lost works.
Sources and further reading
- Alexander, M. (2012). The New Jim Crow: Mass Incarceration in the Age of Colorblindness. The New Press.
- Benjamin, R. (2019). Race After Technology: Abolitionist Tools for the New Jim Code. John Wiley & Sons.
- Bregman, R. (2014). The Case for Basic Income. UBI.Org. https://www.ubi.org/46/ubi-video-rutger-bregman
- Card, O. S. (2017). Ender’s Game. Tom Doherty Associates.
- Delmas, M. A., & Burbano, V. C. (2011). The Drivers of Greenwashing. California Management Review, 54(1), 64–87. https://doi.org/10.1525/cmr.2011.54.1.64
- Dickens, C. (1843). A Christmas Carol in Prose: Being a Ghost Story of Christmas. Chapman & Hall.
- Downey, K. (2009). The Woman Behind the New Deal: The Life of Frances Perkins, FDR’S Secretary of Labor and His Moral Conscience. Knopf Doubleday Publishing Group.
- Eubanks, V. (2019). Automating Inequality: How High-Tech Tools Profile, Police, and Punish the Poor. Picador.
- Get Into The Holiday Spirit With Scandinavian Glogg. (2011, December 22). NPR. https://www.npr.org/2011/12/22/144101579/get-into-the-holiday-spirit-with-scandinavian-glogg
- Harry, S. (2021, January 11). Listening to Black Women: The Innovation Tech Can’t Crack. WIRED. https://www.wired.com/story/listening-to-black-women-the-innovation-tech-cant-figure-out/
- Martin Niemöller: “First they came for the Socialists…” (n.d.). Retrieved February 2, 2022, from https://encyclopedia.ushmm.org/content/en/article/martin-niemoeller-first-they-came-for-the-socialists
- Noble, S. U. (2018). Algorithms of Oppression: How Search Engines Reinforce Racism. NYU Press.
- O’Neil, C. (2016). Weapons of Math Destruction: How Big Data Increases Inequality and Threatens Democracy. Crown.
- Parish, P. (1999). Amelia Bedelia. Harper Collins.
- Plant nutrition. (2022). In Wikipedia. https://en.wikipedia.org/w/index.php?title=Plant_nutrition&oldid=1069256995
- TASAT – There’s a Story About That. (n.d.). Retrieved February 2, 2022, from http://tasat.ucsd.edu/
- Townshend, P. (1971, August 14). Won’t Get Fooled Again—Original Album Version [recorded by The Who]. Decca Records.
- Wachter-Boettcher, S. (2017). Technically Wrong: Sexist Apps, Biased Algorithms, and Other Threats of Toxic Tech. W. W. Norton & Company.
- Zachry, M., & Spyridakis, J. H. (2016). Human-centered design and the field of technical communication. Journal of Technical Writing and Communication, 46(4), 392–401.
Transcript
B | We are super ignorant about mushrooms. |
A | Welcome to TC Talk, where the topic is professional, but the tone isn’t. |
B | Oh heavens, no. So what are we talking about today? |
A | We’re not even done with the intro yet. |
B | What? I am Benton, |
A | I’m Abi. We’re talking about tech comm. |
B | It’s what we do |
A | Unprofessionally. |
B | That’s why I’m here. |
A | Cheers. |
B | Cheers. |
A | Glogg is our drink of choice. It’s a Scandinavian wintertime beverage. |
B | It would keep you warm. |
A | You should know this. |
B | Yah I should. |
A | You were the one who took Norwegian. |
B | [Speaks Norwegian] |
A | How do you say Glogg in Norwegian? |
B | Glogg. |
A | What vowel is that? Is it the O with the dots over it? |
B | Norwegian doesn’t have an umlaut. It does have the null the crossed out O. |
A | That’s what it is. |
B | And that is more of an uh. |
A | Glugg? |
B | They also have the A with the halo which is a more pure O. |
A | That Minnesotan monophthongized O in contrast to a diphthong, |
B | No, a diphthong. |
A | Diphthong. |
B | I actually learned the IPA from a theater guy. He was rigorous about pronunciation. |
A | So is it then monophthong? |
B | I suppose it would be because it isn’t dip, it’s di. |
A | But diphthong sounds like a great insult. So what’s new for you in the technical communication world? In your work safety training book, you found that somebody failed to use keep with next and it drove you nuts. |
B | Oh my goodness, it was ridiculous. It was talking about cold weather hazards. And so the heading was at the very bottom of a page. And then it started talking about hypothermia at the top of the next page with no |
A | Page break, people! |
B | What if I were to start on that page and be like, there’s something above this and then it starts talking about frostbite. Is this also frostbite? What do I do? |
A | Although a good technical writer will not use headings to replace meaningful sentences. So ideally you’d have the heading and then a topic sentence that |
B | includes the word hypothermia. |
A | There you go. |
B | Remember that folks, include hypothermia in the topic sentence of every section. |
A | That would be a pretty cool paper. In my world, I am continuing to read. I even stopped reading a book. Remember how we talked at the very beginning |
B | Oh right, right. |
A | that it’s a challenge for me to not complete a book. And this time I was reading one. And I was like, this is foundational. And it just, I have better things to do. So I closed it. |
B | There you go. One does not need to read all the works of Aristotle. |
A | It wasn’t Aristotle. But I’m not gonna say who it is. |
B | Lest they come after you on Twitter. |
A | I can almost guarantee they’re not on Twitter. They may not even be alive. But the nice thing about that is that it allows me the opportunity to start and finish amazing books. Two of which I want to talk about today. |
B | Great. |
A | Amazing but enraging. As I was reading it, I just kept thinking, this is going to make Benton so mad. |
B | I’m so excited to be so mad. |
A | The first book is by Ruha Benjamin, Race After Technology. |
B | What? Oh, too soon? Too soon. |
A | Yes, don’t get mad yet. Reserve it for when it really counts. Subtitle, Abolitionist tools for the new Jim Code. Published 2019. |
B | For the New Jim Crow? |
A | We’ll get to it. Just wait. And Automating Inequality by Virginia Eubanks, published 2018. Subtitle, How high-tech tools profile, police, and punish the poor. |
B | Some good alliteration, right there. |
A | I actually just noticed that because I’m thinking about all the p plosives that I’m going to have to edit out of this recording. |
B | You just don’t point your face right at, you don’t point your Ps because they pew pew pew. |
A | You redirect your P elsewhere. |
B | Yes, don’t P on the microphone. |
A | I feel like when I said where the topic is professional, but the tone is not, I’m really leaning into that right now. So these authors focus on the effects of technologies on Black people and poor people, respectively. |
B | Okay. So this is going to be about how it lifts them up and supports them and keeps them safe from all manner of discrimination? |
A | No. |
B | No? |
A | You saying that sarcastic question though, it does capture how a lot of these tools are being marketed. Which is, makes me feel like I’m living in backwards land. In our podcast so far, I’ve been, I think, repeatedly emphasizing that rhetoric is not a neutral tool and it’s not inherently bad as the public commonly thinks but even the best, or clearest technical communication is not devoid of bias or ideology. The same is true of technology. |
B | True. |
A | Do I sound like a Luddite? |
B | Well, you’re not calling on the people to wreck the, the looms of 1800s England, so no. |
A | How do you know what the Luddites actually were? See, I was kinda trying to set up to be like, Well actually the Luddites didn’t hate technology just for the sake of hating technology. |
B | They were taking their jobs. |
A | Yes, they hated the human costs of quote unquote progress. And in that sense, I am a Luddite. People in my field, they get that about technology. I don’t think it is quite as widespread of a belief on the outside. I’m thinking of students in my classes, for instance. And this is kind of a new idea for them. I teach a lot of IT students |
B | Oh. |
A | and people who are going into tech need this message the most, right? But the classic sort of argument about the neutrality of tools that is fallacious is from the gun proponents. You know where I’m going with this. |
B | Guns don’t kill people. People kill people. |
A | Well, yeah, but guns make killing people a lot easier. And even things that aren’t purposely designed to cause harm, like guns, can still cause harm. Thinking back to a couple of episodes ago, you gave the example of a lighter. You can use it to create a fire to cook your meal over, or you can burn down California. As professionals, we need to get in the habit of asking the question, What could go wrong? |
B | Merely stated in earnest or stated cheerfully with sarcasm: What could go wrong? |
A | Yes, the tech bros creating algorithms are maybe more blithely ignorant to the actual effects. You may have heard the statistics about how employers discriminate based on the names on a resume. Names that look non-white or female tend to get rejected more. And they’ve done studies on this where the content of the resume is exactly the same and still they show that bias. So it’s a real problem. And in 2013, Amazon had a recruitment tool, the AI, that selected job candidates. It was found that it was discriminating against women. |
B | Hm. |
A | And of course it wasn’t like the programmers built into this algorithm “exclude women.” |
B | It was using data. |
A | The data set consisted of primarily men’s resumes. And so you have to ask those questions. Why was it primarily men’s resumes included in this pool? Well, because of historical sexism, right? |
B | Well these are the resumes of people that we hired. Well, why did you hire them? |
A | I had a conversation with someone about this once where we were talking about this Amazon algorithm. And he was like, but it’s an algorithm. It can’t discriminate. Like what if white men really did have the best resumes? And I was like, I don’t like where this is going. But I said, Well, then you have to ask, what are the structural conditions that make it so that Black people or women have fewer qualifications? Because of other forms of discrimination that may have shut them out from those opportunities. |
B | So fully embedded in the AI is the assumption of meritocracy. |
A | Yes. |
B | Which on its own, sounds good. However, it’s this real fluffy thing when you just talk about it, but when you break it down into measurable things that a machine could understand. It is all the programmer who decides what is deserving of merit. |
A | By the way, the story ended well, the person was like, oh, I see what you mean. Which was unexpected and heartening. |
B | Thank goodness. |
A | But asking the question in a literal sense, what could go wrong? It’s not enough to assume that our products will be used as intended. What could this technology do in the wrong hands or for the wrong purposes? And if we’re responsible for creating a technology, we can get defensive about that. So I understand that’s hard to do. |
B | There is a lot of good dystopian type literature, novels that can help people to see what could go wrong. Because at the very least, someone who knows how to write a novel has thought about |
A | Worst-case scenarios. And maybe that’s a better question to ask, is What’s the worst that could happen? |
B | What could go wrong? How wrong could it go? If this is a line of inquiry that you as a tech writer or perhaps a tech comm instructor would be interested in pursuing, there is a website called TASAT. It’s an acronym for There’s A Story About That, that kind of serves as a database for, you know, like all of these scenarios that have not happened. |
A | If you need practice in envisioning worst-case scenarios, that’s the place to go. |
B | Right? |
A | I don’t need that practice personally. |
B | Right. It isn’t all about worst-case scenarios though. It could be something like Enders Game. |
A | Any contingency. |
B | Where we make contact first and we are the bad guys. And so it’s, it’s more a matter of becoming the worst case rather than encountering it. |
A | People are so wired to place themselves in the shoes of the good guy, |
B | Good person. |
A | Most bad guys, quote unquote, don’t revel in that identity. They wouldn’t self-identify as that. I mean, some, buy |
B | Some do. It’s true. |
A | Like even they have a justification for the choices they make. So I think that’s another thing to do, is stop insisting We’re not bad, we’re not prejudiced. But accept that because we are human, that is impossible. So isn’t the answer to create algorithms that make decisions for us because machines can’t be biased? |
B | We’re not talking about like the, the Terminator series was a happy story about machines coming back to help us through hard times, right? |
A | Let’s get into it. Both authors, Benjamin and Eubanks, introduce phrases that suggest the historical roots of current racist and classist technologies. |
B | Okay. |
A | For Benjamin, her phrase is the new Jim code. And for Eubanks it’s the digital poor house. So let’s break this down. New Jim Code. Let’s back up to what were the Jim Crow laws. |
B | Okay. We’ve backed up a long way. |
A | Yes, but their legacy persists in ways you wouldn’t expect. So racial segregation laws in the South in late 1800s to mid 19 hundreds. So this was post-Civil War, post Reconstruction. And so this was a backlash against, by white people, against Black people for living as humans on equal footing with them or nearing that. So then what is the new Jim Crow? This comes from Michelle Alexander. And she talks about how, that segregation is still happening through racist policing and legal systems. But this time with a colorblind ideology. In other words, laws don’t explicitly refer to race, like we will put you in jail more because you are Black. But because Black people are disproportionately imprisoned, that amounts to a type of segregation. |
B | Yeah. You get to live in the crappiest part of town of all. But it’s free housing. |
A | Oh my gosh, we’ll get to that too. The new Jim code then is a play on that idea that we don’t have the old Jim Crow laws, but we have technologies that continue to discriminate. Harder to see, easier to distance ourselves from because it’s the technology, not us. She defines it as “the employment of new technologies that reflect and reproduce existing inequities, but that are promoted and perceived as more objective or progressive than the discriminatory systems of a previous era.” So the same racist ideologies are simply evolving with the times. |
B | To quote The Who, meet the new boss, same as the old boss. |
A | And these technologies can claim to be race-neutral. But in so doing, it can cover up the racist consequences. You may not be creating an algorithm that targets Black people. But because of past segregation, zip codes are a really reliable proxy for race. Now, let’s move on to the idea of digital poor houses. |
B | Okay, |
A | What do you know about poor houses? |
B | I believe that poor houses were preceded by what you see in a Christmas Carol, Charles Dickens’ story, where Scrooge’s parents went to debtors’ prison. Because they couldn’t pay their debts they went to a jail for people who couldn’t pay their debts. And so, in a sense, |
A | A poor house is a little bit more charitable than that. |
B | It’s a little bit more charitable than that. You know, it’s, it’s showing a modicum of reform. |
A | Speaking of A Christmas Carol. As I was reading Eubanks’ book, I couldn’t help but think, we are Ebenezer Scrooge, like the middle-class, |
B | Me? |
A | The upper-class. No, I’m serious. Scrooge is meant to be this odious character, right? And yet, if you look at the realities of poverty in the United States, are you mad yet? |
B | I’m starting to simmer a little bit. Dickens obviously was a great author. He picked up on, this is what capitalism does to people. It reduces their value to the value of their money. There’s no room for human dignity in GDP. Somehow they managed to get reform to happen. And there were legal protections for people. They abolished debtors’ prisons. But I would like to hear your end of it. |
A | In the United States, poorhouses started to become a thing in 1800s. New York specifically is where this kinda kicked off. We’re giving poor people a place to live. What could go wrong? People got contracts from the government to care for the poor. So they were paid per quote unquote pauper. But then they could use the residents’ labor as a source of profit for themselves. So, people living in a poor house, often, at least able-bodied people in a poor house often had to participate in farming, laundry, working in a quarry, et cetera. And inmates had to sign an oath essentially stripping away their rights for things like voting, getting married, holding office. |
B | Geez./ |
A | She writes, “poorhouse proponents reasoned that the institution could provide care while instilling moral values of thrift and industry.” |
B | That sounds very Reagan era. |
A | So in these contexts, I mean, it hastened death in a lot of cases. And that belief, that part of the way to eradicate poverty is to eradicate the poor. |
B | Geez. That’s a line right out of Christmas Carol. |
A | Decrease the surplus population. |
B | Exactly that. |
A | Conditions in poor houses were generally pretty awful and that started to get noticed. Plus in the 18 seventies, there was an economic depression. More poor people than ever before. And in larger numbers, those poor people had some power that was scaring the upper classes. That was around the time that Franklin Roosevelt proposed some progressive reforms like the Social Security Act. I don’t know if you know this history of it, but he made a compromise because he was afraid that the Southern states wouldn’t sign-on. And so they excluded domestic and agricultural workers from the protections afforded by this Act. So that predominantly harmed African Americans. |
B | A lot of people on the political left in America raise FDR up as, as an example of greatness. I mean, he got us through World War Two. He put out the new deal and people loved him so much, they elected him to death. There are some racist things in his past, like the Japanese internment camps during World War Two. This. People want to think that he was this great guy pushing for social reforms when really, it was all Frances Perkins, the New Deal was all her. |
A | Who’s that? |
B | Frances Perkins was his Secretary of Labor, the first female Secretary of Labor. I got this book about her life. It’s titled The Woman behind the New Deal, The Life of Francis Perkins, FDR’s Secretary of Labor, and his Moral Conscience. And it was written by Kirstin Downey. |
A | Thank you. |
B | She was a champion of the poor for numerous years. And she did some of, some of her work in New York City, which got her connections to the elite, which is eventually how FDR came to know her and asked if she would come and work as his Secretary of Labor. She laid down an ultimatum, If we don’t do this I’m on not on board. And he was like, All right. |
A | This meaning what? |
B | Minimum wage, 40 hour work week, Social Security. She twisted his arm into doing it. |
A | And that’s going to be the case. Any movements on behalf of the people are going to require |
B | Arm twisting. Because the powerful won’t relinquish power willingly. |
A | After poor houses, we moved into the age of scientific charity. Instead of just putting everyone in these poor houses regardless of whether they, quote unquote, deserve it, we’ll use data-driven methods to figure out who really needs it and who doesn’t. This is when casework became a thing. Caseworkers started to make individual determinations about whether somebody deserved welfare. As you can imagine, this involved a lot of bias and it was very invasive for the families involved. For instance, |
B | It is still very invasive for the families involved. |
A | We’re getting there. Listen to this. “In 1963, caseworkers in Alameda County, California invaded the homes of 700 welfare recipients one cold January night, rousting mothers and children from their beds in an attempt to uncover unreported paramours.” They didn’t identify themselves. They shouted at them, they broke down doors. That’s terrifying. |
B | That’s state-sponsored terrorism, in fact. |
A | That was ugly and starting to get attention. And the welfare rights movement gained a lot of ground in the 1960s. And so the technologies of poverty management had to evolve with the times as well. |
B | Management, not alleviation. |
A | You nailed it. |
B | Fuck. |
A | The digital poor house, then, represents those ways of managing the poor through surveillance and automation and tracking. We, as a country wouldn’t stand for Jim Crow laws or poor houses today, you’d hope, because they’re too overtly discriminatory. But the modern iterations of these ideologies in the form of technologies are almost worse because they’re sneakier and because they are often marketed as efforts at diversity and fairness because you’re outsourcing the decision-makers to a machine instead of biased humans. What could go wrong? |
B | What could go wrong? |
A | So maybe the creators of this technology were naive and idealistic and really thought that their product was going to lift the poor out of poverty or end racism or whatever. Or maybe they were just using the language of social justice to get people on board with their product. And frankly, if the impacts are discriminatory, it doesn’t matter whether or not you intended that. I love what Eubanks say here, “automation and analytics do not remove bias. They launder it, performing a high-tech sleight of hand that encourages us to perceive deeply political decisions as natural and inevitable.” |
B | Like greenwashing. But for race. |
A | What’s greenwashing? |
B | Oh, that’s when Exxon and Shell and all of those oil companies say, Look at what we’re doing to save the environment. |
A | Oh, to kind of draw attention away from emissions. |
B | What their core business does. Yeah. Anything that you see on the Twitter account of any oil company, it is textbook greenwashing. That’s all it is there. |
A | Because it makes you go, Oh, they acknowledge this is a problem, awesome. |
B | They’re not so bad. And ignoring the fact that 99.5% of what they do, having oil spills here, there, everywhere, hundreds a year, pipelines, derricks, you name it. Everything they make leaks. |
A | But they gave the ducklings a bath. |
B | In Dawn. |
A | The dishwashing soap. |
B | And now Dawn is somehow the good guy? And they’ve run commercials where volunteers are cleaning up oil-soaked ducks with Dawn. |
A | Benton. That’s why I buy Dawn dishwashing soap. |
B | It’s greenwashing. |
A | But it’s cute. |
B | I suppose, in the case of Dawn it is actually blue washing. |
A | Because it’s blue soap. I see what you did there. But honestly forget ethos, pathos and logos. If you want to sell me something, put a cute animal on it. What’s the name of the rhetorical appeal for that? |
B | Cuthos. |
A | I like that. |
B | It’s from Aristotle’s unknown works. Cuthos. |
A | His apocryphal works. Let’s get into some examples. |
B | Do it. |
A | We know that the prison industrial complex sucks. |
B | We know less well than people who know from experience, but we do know. |
A | Yes. And we know that Black people are disproportionately incarcerated |
B | Dragged into that system, yep. |
A | So a tech solution might be, well, let’s stop putting people in prison. We’ll just use ankle monitors. |
B | What could go wrong? |
A | Sure, maybe people aren’t inside cells, but they’re not out of the system. And it becomes easier to deploy on a larger scale. |
B | Someone’s gotta design the ankle monitors. Someone has to produce them. There are workers involved, there’s money to be made from government contracts. |
A | Exactly. Exactly. And yet if you can slap a label on it, “this solves the problem of mass incarceration.” Then yes, the green washing. Except in this case not greenwashing, justice washing. I don’t know. |
B | Not only do you have the product going into that, you’ve also got the data coming out of it. Can you imagine what it would look like if you mapped out a day or a week of where everyone in a town with these ankle monitors is? |
A | That would be valuable data. |
B | And who would have access to it? |
A | The company. But then they can sell it to other companies. |
B | I imagine like they would sell that to the police or provide it as a part of their service. And can you imagine like a density map of how often convicted criminals are in a location. |
A | This is a perfect segue to the next technology I’m going to talk about, predictive policing. |
B | Exactly. |
A | The idea here is that “we can keep neighborhoods safer by directing the police resources where they’re most needed.” But again, it’s an algorithm that determines where the quote, unquote dangerous areas are. So why are those neighborhoods predicted to be higher crime? Probably based on past arrests, |
B | Which is based on race. |
A | Making things worse, with these predictive algorithms, officers will then be going into these locations with an expectation of more crime. |
B | They’re looking for someone to bust. |
A | So they will make more arrests and that data gets fed back into the system. |
B | It’s a positive feedback loop. |
A | And in talking about algorithms with my students, I like to use the metaphor of a recipe. |
B | A recipe? |
A | Yeah, because an algorithm, at its essence is a set of instructions to create some output based on some input. So think of all the ways that a recipe can go wrong, either in the writing of it or the following of it. |
B | Yes. Amelia Bedelia’s cookbook. |
A | To those of you who aren’t familiar with the children’s books, Amelia Bedelia, she’s a housekeeper. She takes everything literally. |
B | When she’s told draw the drapes. She’s like, I don’t know why I should do that, but she gets out a piece of paper and draws the drapes. |
A | Dress the turkey, selects an outfit for it. Yes. So the interpretation of the recipe. The recipe itself could just be shit in the first place. Can you think of an example of a recipe for something that should not exist in the world? |
B | Oh, okay. Where the intended output is not worth making? |
A | Yes. |
B | Peanut butter and barbecue sandwich? |
A | That’s a literal thing that you have made and eaten in your lifetime. |
B | It wasn’t as bad as you’d think. |
A | Let’s not forget your spaghetti sandwich. You’ve come a long way in your cooking skills. I’ll put it that way. |
B | It isn’t, Okay, the spaghetti sandwich, that was not a cooking skills thing. That was I need to slap together a lunch and here is what’s in the fridge, done. |
A | But now you’re making mirepoix. |
B | Yes, it is very delectable. |
A | So you can make a recipe for a spaghetti sandwich, which should not be made in the first place. But let’s assume that someone has good intentions. |
B | You could make it out of garlic toast and then it would be more reasonable. See, it just needed to be workshopped. |
A | You know what though, I am guilty of seeing weird recipes and being like those two things should not go together. So there must be something behind this so I must try it. Sauerkraut pizza. That wasn’t even a recipe. I just did that. I kind of modeled it after a Rueben sandwich though. |
B | It was pretty good. |
A | It was. |
B | I do remember there was the time where you made that. What was it? It was like a crock-potted parsnip and wild rice something that was not quite right. Something was wrong. |
A | The wild rice expanded and was like popping out of the pot. |
B | I think there’s a special way to do wild rice in a crock-pot and you did not do that. |
A | Why did we even have parsnips? I don’t want to knock parsnips. I just don’t know how to prepare them. |
B | Yeah, apparently there’s an outer rind that needs to be discarded because it’s bitter. I didn’t know that until this last |
A | That’s any kind of parsnip or wild parsnips? |
B | They are the same plant. |
A | Oh, okay. |
B | I remember struggling through it for days. Eating leftovers. Because that’s what I do. I cannot waste food. |
A | Our daughters call you our family garbage disposal. Because if I cook something awful |
B | Because I eat garbage? |
A | you will faithfully eat it. We’ve come a long way. |
B | Yes, we have. We’ve both gotten a lot better at making food. And I am just as good at eating food, if not better. |
A | Alright. The ingredients, maybe the ingredients do not add up to the thing you want to make. |
B | There’s times where you use an ingredient in multiple different places. And it’ll say like butter, separated. Separated how? |
A | Yes. So the ratios of things, I’m trying to get us back on track with this metaphor. |
B | Oh, you are, I’m sorry. |
A | Substitutions. You don’t have whole milk so you substitute sweetened condensed milk or something, which would probably make it better to be honest, whatever the thing is. And that’s kind of like these proxies. If you’re going to predict something, then your data comes from past trends, past arrest rates. And maybe you’ve got a recipe for a good thing, chocolate chip cookies. And then people use the chocolate chip cookies as projectiles to harm their neighbors. I don’t know. |
B | They, they slip in a little arsenic and clear out the neighborhood. |
A | Don’t try this at home. |
B | Don’t try this anywhere. |
A | Anyway, the idea of a recipe I find helpful. There’s a lot of different points along the way where things can go wrong and leave you with an undesirable product. And the thing about algorithms, unlike recipes, is that they’re usually much more opaque. And people inherently trust them more because technology, machines, data! Right? So those are some racial fixes that had unintended consequences. Or intended. Perverted. Okay, and then in automated inequality, like I said before, this focuses on technologies of poverty management. She says, “we manage the individual poor in order to escape our shared responsibility for eradicating poverty.” Eubanks gives very specific real life examples of technological systems that were put in place. The first one she talks about was an automated welfare eligibility system put in place in Indiana in 2006. And the problem that that was responding to was supposedly there was some welfare fraud going on and the Republican governor at the time really seized upon that to push this new approach to welfare. And they argued it would be more fair because it took the case workers out of the equation. And case workers are human and therefore, bias, right? |
B | What could go wrong? |
A | Well, in order to determine who’s eligible, applicants had to fill out this extensive application. And if they missed one thing or one technicality, then the system would automatically reject them without telling them why. People would send in their legal documents, birth certificates, driver’s licenses, and would not get them back. They would just go to this black hole essentially. I am sure that my blood pressure was rising as I was reading this chapter. So essentially the system ultimately rejected like a million requests for benefits. |
B | From what state? |
A | Indiana. |
B | Indiana? |
A | The number of people on the welfare rolls was drastically cut. Here’s another problem. |
B | To make it more fair, nobody will get anything. |
A | Basically. Okay, poor people have less reliable access to the Internet and other technologies required. If they tried to get, they tried to talk to someone at the call center, they |
B | probably had to wait on hold in a queue for hours because there were a million people who needed help. |
A | It may not have been a million people. It was a million denials. I don’t know how many per person, but the city graveyard was complaining about not being paid for the funerals and burial of poor people. Are you mad yet? The thing about this whole system is that it actually worked as intended, because the intention was to knock people off of welfare. A lot of the errors that people ran into. |
B | That’s what they were going for. |
A | Yeah. It wasn’t a bug. It was a feature. It was meant to be onerous. The situation didn’t start to turn around until poor people started organizing and the media started to pay attention to specific cases like young children getting kicked off of their health insurance or nuns stuck in the hospital worried about paying a bill. Little old ladies, whatever. The point is that the media had to tell a story. And that story had to focus on the deserving poor. They had to paint a picture of innocent people who were being harmed by this. And that’s, it lead to action. The public paid attention. But that is not a sustainable way to portray poverty in our country, as either people deserve it or they don’t. Because every human has a right to dignity. |
B | Yeah, deserving is not a universally agreed upon definition. |
A | Yeah. How about this. Skid Row, LA, very high homeless population. And the process of getting people connected with housing was, for a long time, not super regulated. And so obviously discrimination could come into play there. Again, it’s difficult to separate the truly vulnerable from those who are not. Those who deserve housing versus who don’t. Again, this is all in the logic of capitalism. So here’s the tech solution. Create a database that matches people to available housing according to their need. And this was implemented |
B | According to their need. You’re homeless. You don’t need a house. |
A | That was actually what it came down to because there was limited housing. So they created this algorithm to assess people’s vulnerability to prioritize the housing that was available. This was called coordinated entry, started in LA in 2011. What could go wrong? |
B | What could go wrong? |
A | Applicants had to fill out the application multiple times. And this did not guarantee housing. And they had to assent to invasions of privacy. They had to give up health information, immigration status, substance abuse, even where they were at different points of the day. Little did they know that this data was shared among 168 organizations. And here’s the worst part. Law enforcement was one of the recipients. And they were, are perhaps able to access the data at any time. Listen to this from the book. “There’s a long history of social services and the police collaborating to criminalize the poor in the United States. The most direct parallel is Operation Talon, a joint effort of the Office of Inspector General and local welfare offices that mined food stamp data to identify those with outstanding warrants, then lured them to appointments regarding their benefits. When targeted recipients arrived at the welfare office, they were arrested.” |
B | I’m mad. |
A | She calls this new surveillance. Whereas old surveillance, she said, “the target had to be identified before the watcher could surveil.” But with access to big data, you can then analyze that data. |
B | It’s not personal. |
A | And targets will kind of float to the top. This tech solution was meant to solve the problem of limited housing available in LA. But it didn’t actually result in new housing. It just dehumanized the process of deciding who gets the existing housing. |
B | There was a study in, I think it was in London. They were looking at how to get people housed who were, as they say in England, sleeping rough. They had one social worker there who was like working with them to find like a holistic, what can we do to improve your situation? Found in a lot of cases that what they needed was not a lot of money. Frankly, what they were being paid to administer this program was substantially more than these people needed. |
A | So just give people cash. |
B | Give people cash. |
A | Yes, that’s actually one of the solutions that Eubanks suggests is a universal basic income, right? |
B | Great. |
A | Cash, no strings attached. |
B | Because you get rid of all that administration. You don’t need to worry as much about fraud. |
A | Although I want to be cautious because we have to ask |
B | What would go wrong. Absolutely. |
A | That being said, the kind of root assumptions behind universal basic income are quite different than the idea of sorting the deserving from the undeserving. |
B | Assumptions and goals are dramatically different. |
A | All right, how about this? This is a real problem, child abuse. |
B | That is a real problem. |
A | The CDC estimates that one in four children will experience some form of abuse or neglect in their lifetimes. So what do we do about it? In Allegheny County, Pennsylvania, they used a child abuse prediction algorithm. |
B | Oh boy, what could go wrong? My guess is that it would predict based on demographic reasons, who would be likely to be child abusive, and then people who are good at heart parents and not abusive, but just living in poor conditions, are under greater scrutiny. I have a feeling that there’s both the predictive policing conundrum, looking in certain areas more than others, more likely to find false positives. |
A | You’re good at this game. |
B | It’s not a game that I like being good at. But |
A | Yes, one of the ingredients in this algorithm, for instance, was the number of times a certain family gets reported to the Child Welfare offices. But the reporting process is anonymous. And who is more likely to get reported? So Black and bi-racial families were disproportionately targeted that way. And that’s an ingredient that goes into the recipe, right? How about this? Their database only consists of data from people who accessed public services. |
B | What. |
A | Any kind of public assistance. |
B | Okay. So you’re automatically turning a blind eye to rich abusers? |
A | Yes. |
B | Fucking A. |
A | But wait! |
B | There’s more? |
A | One thing that they did when using this algorithm that was thoughtful was that they didn’t leave the algorithm to assign the final risk score. For every case, there was also a human intake screener. Wouldn’t that be the best of both worlds? |
B | Or the worst of both worlds. |
A | Combining human judgment with algorithmic decision-making? The existence of this rating, it causes these workers to question their own judgment. |
B | And I’m sure that a part of the, having had reports is say you’ve got a kid who has narcolepsy or something like that, something that makes them extremely accident prone. And so they show up to school with, with bruises. And because the family is poor, they don’t have the means to give them, get them the help that they should have. They’ve tried to get on public assistance. And so now they’re on the radar of this god awful system. And they’ve got a high score. They love their kid but they can’t get the social security to help them. They can’t get the this. They can’t get the that. |
A | Yes. Again, they have to trade dignity and privacy for access to the services. Which again, that reflects the underlying ideological belief about poor people. That they’re not inherently deserving of privacy and dignity. |
B | Right. Human rights for all who can afford it. |
A | Yeah. Benjamin says that we shouldn’t be surprised that old bias is coded into new technologies. But we will be surprised if we believe that technological progress just naturally equates to social progress. It does not. |
B | Right. It’s the myth of progress. |
A | Let’s talk actual solutions, not just ill-conceived technological fixes. |
B | What could go wrong? Oh, wait, are we done with that part? |
A | Yes. |
B | Okay. Sorry. |
A | Well, you can’t solve systemic problems by only treating the symptoms. And you can’t change underlying attitudes with a tech tool. We have to actually change the way that we think about and consequently how we talk about race and poverty. In that sense, one solution is rhetorical rather than technological. Like I mentioned before, how the media tells stories about the deserving poor versus the undeserving poor. That is problematic. That is something that rhetoric scholars should be aware of and intervene in. That’s what Benjamin says, is tell stories. As a contrast to data points. So the popular saying is, anecdotes do not make data, but data are also not anecdotes, you know. The word anecdote for people in science and people who value research is almost thought of dismissively. But people’s stories, like individual stories, do matter, you know? We need to acknowledge the intricacies, or that intricacies exist for every human in these systems. |
B | Yeah, I think that it’s a super pervasive thing to oversimplify in modern society, like just because life is so complex, you know, the scientific method, you want to isolate things as much as you can. And so early on in the scientific study of agriculture, they discovered the three bulk nutrients for plants, nitrogen, potassium, and phosphorus. Because we had that, then people are like, that’s all that matters! When that is not all that matters. |
A | There’s a lot more going on. |
B | I mean, even if you start looking at other components of nutrition for plants, you’re still missing it because there’s the, the entire interconnected ecology. But you are never going to disentangle all of that. Like we, we know so very little about just fungus. And the crucially important role that it plays in our ecosystem. We are super ignorant about mushrooms. Mushrooms, just the way that like fungus grows in all these tiny fibers that grow together. It’s just, it’s very difficult to even try to study it. |
A | Here’s the thing though. I get that desire to simplify complexity. And it’s, it’s something that any researcher does, even a qualitative researcher, they still have to derive patterns from complex data. |
B | It’s an evolved, it’s an evolved mechanism. Imagine someone who is in a hunter-gather community. People who recognize the pattern of like, it gets really quiet when there’s a predator lurking around, those people survived. |
A | Patterns are not inherently bad to look for either. It’s just that what happens with these algorithms is that they’re reverse engineering something that is infinitely complex. So the big solution would be to actually understand the problem because a lot of these are solving the wrong problem, right? Managing poverty, not alleviating it, |
B | Or eliminating it even. |
A | Right. But if there is to be a tech solution, then that technology has to be built deliberately anti-racist. |
B | Agreed. |
A | It’s not enough to be neutral. It’s not enough to be apolitical. And it’s not even enough to claim that your product enhances diversity or equality because discrimination is the default. So as Eubanks says, designing for neutrality is designing for the status quo. And those who are doing the designing, the status quo happens to work for them in most cases. And so they’re not used to interrogating those things. In technical communication, UX, user experience circles, human-centered design has been an important concept. Along with that, we have to ask which humans are we centering? And whether that centering is just or unjust. Maybe centering a certain population is the problem in the first place. Like the child abuse algorithm. The, you’re centering people to surveil them. And another good way to think about which humans are being centered is to ask, would the tool be tolerated by non-poor people? She gives an awesome example of this. Somebody made a white-collar crime early warning system. |
B | Oh, wow. |
A | It’s modeled after predictive policing. But instead it focuses on who’s most likely to |
B | Embezzle or |
A | And so if you were to try to put together a composite, stereotypical criminal in this case it would be, you know, white man. Would we tolerate that? |
B | The only white-collar criminal I know is a white man. And he is in spa jail, which makes me angry to no end. |
A | Spa jail? |
B | You know who I’m talking about, right? |
A | Yes. But there’s a spa at his jail? |
B | It’s a white-collar criminal penitentiary. |
A | And it’s kinda like a summer camp is what I heard. |
B | One way to look at it is how would you feel being processed by this system? |
A | Oh, yeah. |
B | How would I feel being identified as a probable white-collar criminal? |
A | You wouldn’t like it! |
B | Would I like having to submit everything about myself and where I am, the answer is no. |
A | Yeah. And again, to ask the question, what’s the worst that could happen? And because that is so impossibly hard for creators themselves to ask honestly, have third parties do equity audits. An example, that child abuse prediction algorithm was initially going to be rolled out in New Zealand. |
B | Okay. |
A | But it did not pass ethical review. |
B | Go figure. |
A | People got up in arms about it. And so it is not happening in New Zealand, but the United States bought it. |
B | We have a product of inferior quality. Where can we bring it? I know, the US. |
A | Obviously transparency of the algorithm. What data is being fed into it, what assumptions are being made? That’s important. Giving people control over their own data is important. That’s really hard to make happen in a capitalist society where people’s data has so much value to corporations. |
B | And so much of our legal system is centered around protecting corporate rights. |
A | Yeah. Involve the group that will be targeted by the technology. These are all good things. But even that child abuse prediction algorithm we talked about, its design was participatory and transparent. And that didn’t solve the root problems of it. So I don’t mean to say that, that that’s a foolproof solution. And maybe we should ask, do we need to collect data at all? It’s not enough to just trust that these tons and tons of data points will not be used in malicious ways in the future. Again, intent doesn’t matter. Maybe someone’s intent right now is perfectly innocent, but that data is there. |
B | When you said, Do we need to collect data at all? I just imagined so many tech optimists, pearl clutching like |
A | So advocating for strong data protections or data privacy laws. Like I was talking about before, with social media and misinformation. Yes, there is benefit to us as individuals knowing how to recognize it, but we also need to try to address the problem at the level of policy. Technology is political. Benjamin says, “machine learning systems in particular, allow officials to outsource decisions that are or should be the purview of democratic oversight. Even when public agencies are employing such systems, private companies are the ones developing them, thereby acting like political entities, but with none of the checks and balances.” Are you mad? |
B | Yes. |
A | Solidarity. Support the organizers, the people who are organizing on behalf of these marginalized groups. |
B | Yes. |
A | Find the movements that they’ve started and join in. |
B | You had said solidarity. I would take it a step further. So I wanted to kind of bring up a difference that was pointed out by the creators of Crazy Town, which is my podcast of the moment. So one of the things that they brought up is the difference between support and solidarity. So support is going to a rally showing up, being there and then going home. When you go home at the end of it, you don’t worry if you’re going to be targeted. If you’re going to have violence inflicted upon you. Solidarity is, is like a demonstration of not being a fair weather supporter. |
A | You may feel safely ensconced in your middle-class existence. But the boundaries are more porous than we think. The tools that are used on the poor today or the tools that are used on Black people today. They’re going to be used on us. |
B | That digs right into that great neighbor quote. When they came for the, it starts like that |
A | Yeah, and I mean, it kind of taps into that self-interest. And if that’s what it takes, is worrying about yourself, to step in and try to protect others. Although we should want to do it out of the goodness of our own hearts and our desire for a just society. And then a caution for people in my field. And this is mostly a caution to myself, I guess, is to not automatically jump to technological solutions. I’m collecting data for a research project right now that is based on a problem that I want to fix in the world. And I confess, my first thought was oh, we could make an app for that. |
B | What could go wrong? |
A | And to be fair, the data consists of talking to people who would be the recipients of whatever intervention. And the first phase is not about proposing solutions at all. It’s figuring out what is the problem and what do people need. And then we’ll see what emerges out of that. |
B | Sounds properly investigative. |
A | So this is heavy stuff. I think these would be totally appropriate to use in the classroom. I think |
B | These? These what? |
A | These books. |
B | These books. |
A | That we just talked about. |
B | Oh, yes. Yes. Those books. |
A | The two books floating above your head, I don’t know. I think students need to know about this, especially undergraduates going into STEM. If you wanted to make a course out of it or a unit out of it. There are a bunch of other really great, readable, thought-provoking books that address this topic one way or another. Those are Algorithms of Oppression by Safiya Noble, Weapons of Math Destruction by Cathy O’Neil. |
B | That’s math, M A T H. |
A | Yep, and Technically Wrong by Sarah Wachter-Boettcher. I’ll put those on our website. The website is faculty.mnsu/tctalk. that’s M as in Mary, N as in Ned. What are the alpha tango whatevers for M and N? |
B | You mean the NATO International Phonetic Alphabet? |
A | Yes. |
B | Mike and November. |
A | Oh, okay. Speaking of November, you can prevent hypothermia by staying indoors on cold days. |
B | Or being a mushroom. |
A | But do we know that about mushrooms? Whether or not they can get hypothermia? There’s a lot we don’t know about mushrooms, remember? |
B | That’s true. That’s true. I’ll try to remember to ask the next mushroom I meet. |